Main Second Level Navigation
Breadcrumbs
- Home
- Education
- Professional Development Opportunities
- Explainable AI: How to create deployable AI in Healthcare
Explainable AI: How to create deployable AI in Healthcare
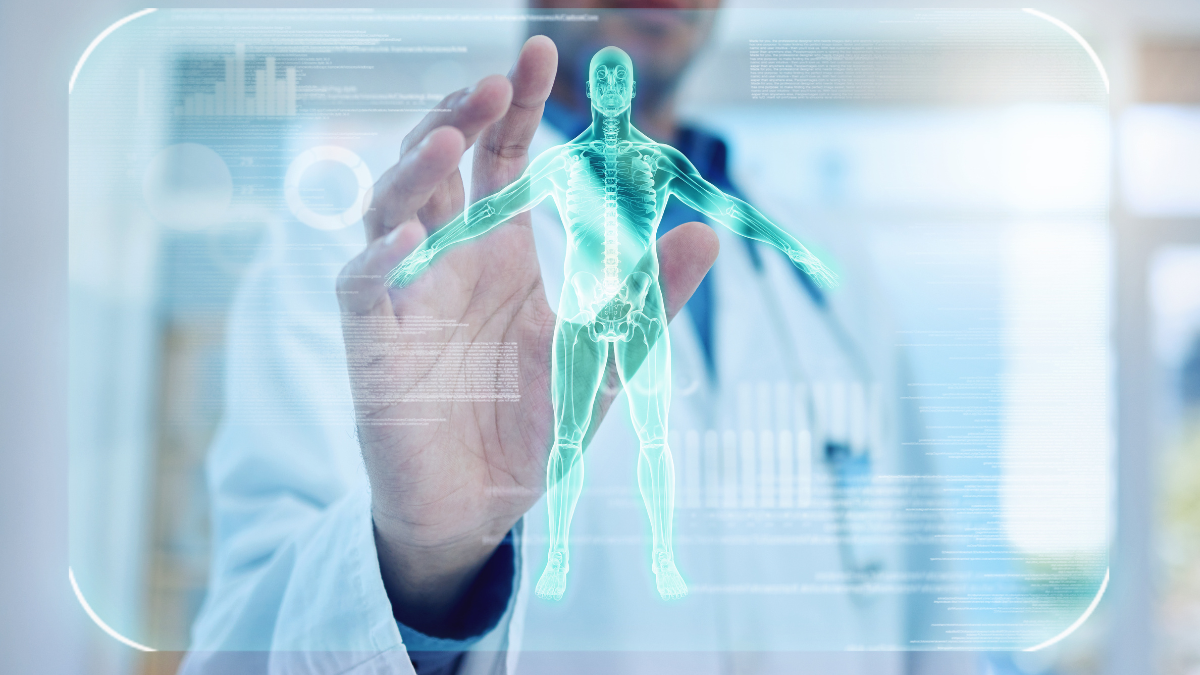
Overview
Join us for a hands-on tutorial that bridges the gap between AI development and real-world clinical application. This session will guide participants through the critical components of building explainable, trustworthy, and clinically deployable AI models. From identifying actionable clinical needs and aligning predictive timelines with medical workflows to selecting interpretable models and designing clinician-friendly interfaces, this tutorial emphasizes practical strategies for successful AI integration in healthcare. Participants will also explore powerful explainability tools like SHAP and LIME, and learn how to communicate AI insights effectively to clinical stakeholders. Whether you're developing a single predictive model or planning a comprehensive multi-model dashboard, this tutorial offers essential guidance for creating AI solutions that clinicians will understand, trust, and use.
Workshop Description
Artificial intelligence (AI) is increasingly being integrated into healthcare, yet many AI models fail to transition from research to real-world deployment. This gap often arises due to misalignment with clinical needs, lack of transparency, and poor integration into clinical workflows. To bridge this divide, AI models must be explainable, actionable, and designed with the clinical need and end-users in mind.
This tutorial addresses the critical need for clinically oriented, explainable AI (XAI) in healthcare, equipping participants with the skills to develop models that clinicians can trust and use. AI adoption in medicine requires models that not only make accurate predictions but also provide interpretable insights that align with medical decision-making. Without transparency, even high-performing models risk rejection by healthcare providers due to concerns about reliability, bias, and ethical implications. Furthermore, clinical workflows operate within specific time constraints, requiring AI models that use available information and provide timely predictions within an appropriate decision-making window.
This session will guide participants through the key considerations in developing deployable AI, from selecting the right model to integrating multi-model dashboards for enhanced and practical clinical decision support. By fostering collaboration between clinicians and data scientists, this tutorial aims to promote the development of AI solutions that are not only innovative but also practical, explainable, and clinically impactful.
Workshop Topics
This tutorial will focus on the key considerations for developing explainable AI and communicating AI model to clinical end-users. We will include the following topics:
Identifying an actionable clinical need: Learn how to define AI problems that address real-world clinical challenges and provide measurable impact on patient care.
Determining the modeling time-window for clinical workflow: Explore how to align predictive modeling with clinical decision-making timelines (recognizing when and how information is available) to ensure timely and useful AI outputs.
Selecting an appropriate AI model: Understand how to choose AI models that balance predictive accuracy, interpretability, and feasibility for clinical deployment.
Explainable AI: Discover key techniques such as SHAP, LIME, and counterfactual explanations to enhance transparency and trust in AI-driven healthcare decisions. Consider how these insights can and should be communicated to clinical stakeholders.
Clinician-facing model interfaces: Learn best practices for designing AI tools that integrate seamlessly into clinician workflows, ensuring usability and adoption.
Thinking big: multi-model departmental dashboards: Explore how to develop AI-driven dashboards for clinical departments that combine multiple models and data streams for comprehensive clinical decision support. Recognize the value in beginning these consultation processes prior to development / deployment of an individual model.
Session Timing
The tutorial will be part of the AIME 25 conference in Pavia, Italy, from 23-26 June 2025.
Learn more about the Conference tutorials
Tutorial Chair
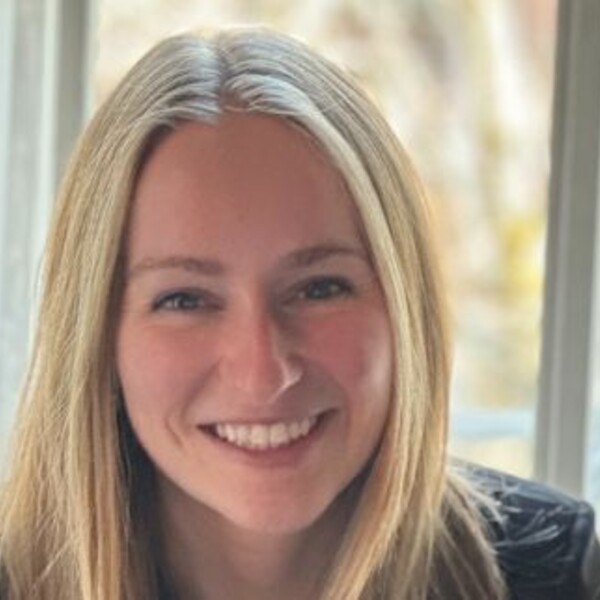
Gemma Postill
Gemma’s research with expertise lies in healthcare AI applications for outcome prediction and clinical decision support. She also has expertise in medical education, having led multiple initiatives on AI literacy for healthcare professionals and is actively involved in research on AI competency frameworks. Together, the research and education initiatives she leads help to bridge the gap between AI development and real-world clinical implementation.
Program Committee
Laura Rosella, PhD
Professor, University of Toronto
Education Lead, Temerty Center for Artificial Intelligence Research and Education in Medicine, University of Toronto
Rahul G. Krishnan, PhD
Professor of Computer Science, University of Toronto
Abhishek Moturu
Computer Science PhD Candidate, University of Toronto
Student Education Co-Lead, Temerty Center for Artificial Intelligence Research and Education in Medicine, University of Toronto
Julie Midroni
MD Candidate, University of Toronto
Education Affiliate, Temerty Center for Artificial Intelligence Research and Education in Medicine, University of Toronto
Vinyas Harish, MD, PhD
Anesthesiology Resident, University of Toronto