Main Second Level Navigation
Trainee Rounds: Suraj Bansal and Sidrah Laldin
DATE: June 25, 2025 (Wed.)
TIME: 12pm to 1pm ET
PRESENTERS: Suraj Bansal and Sidrah Laldin
Learn more about the presenters
Suraj Bansal
Title of Talk
ATLAS-AML: An automated bioinformatics pipeline for drug target characterization and discovery in acute myeloid leukemia
Description
Acute myeloid leukemia (AML) is a heterogeneous disease with poor survival outcomes. An important checkpoint for AML drug development is identifying targets enriched on leukemia stem cells (LSCs) without perturbing normal hematopoiesis. However, drugs satisfying this criterion face additional challenges attributable to heterogeneity in AML wherein adverse genomic drivers and LSC-enriched cellular hierarchies cause chemoresistance and relapse. To facilitate translational efforts in AML, we must ensure target candidates are both enriched on leukemia cells and associated with sources of AML heterogeneity. To bridge this gap, we introduce ATLAS-AML, an automated bioinformatics pipeline for transcriptomic meta-analysis in adult and pediatric AML.
Sidrah Laldin
Title of Talk
Comparing innovative AI algorithms to assess echocardiographic videos for clinical modelling
Description
The expanding role of machine learning in cardiovascular imaging has ushered in an era with the myriad imaging modalities being assessed for hidden repositories of information. Transthoracic echocardiography, being the most common and accessible diagnostic modality to assess cardiac function and structure, serves as the backbone to cardiovascular imaging. Despite its critical role, there are limitations in its use including variance in human interpretation of images leading to the lack of precision and reproducibility. Although there is a rise in using machine learning models on echocardiograms, the research has been limited to still image-based methods, precluding the information that can be extracted by longitudinal analysis of medical videos, the inherent format of echocardiography. We conduct a comparative study that employs the use of multiple dynamic deep learning algorithms to develop predictive models with video-based echocardiographic images using sample size determination as a key variable to assess optimal performance metrics.
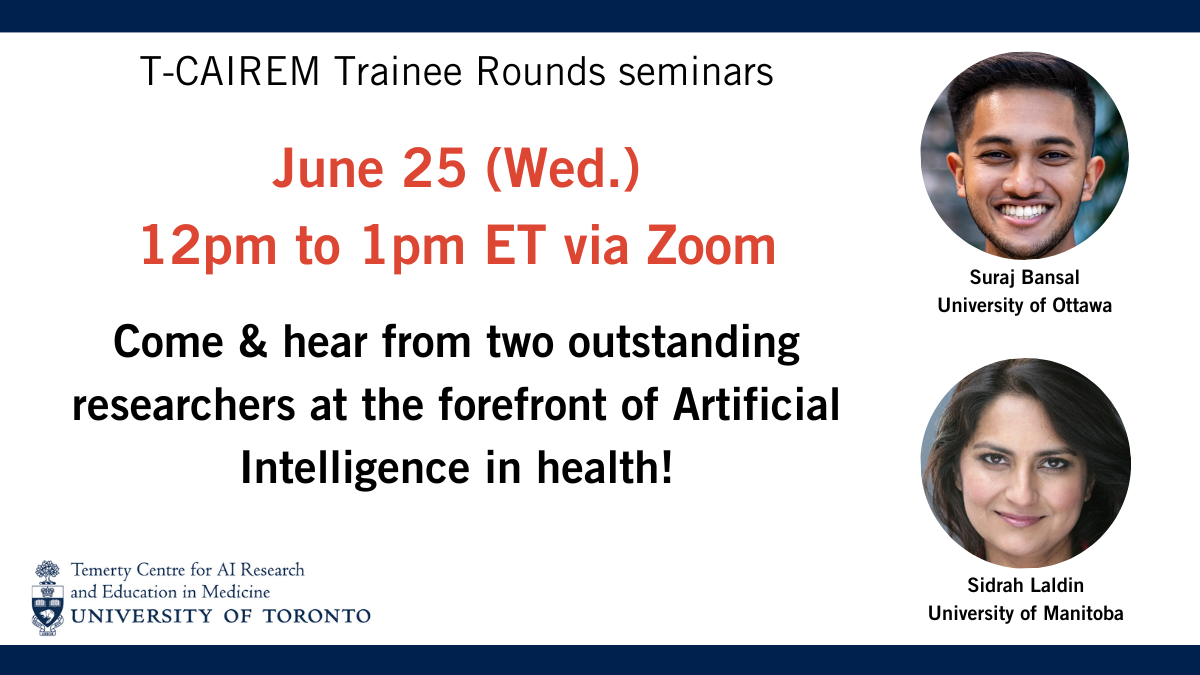