Main Second Level Navigation
Trainee Rounds seminars: AI in Medicine
DATE: June 1, 2021 (Tuesday)
TIME: 12pm to 1pm
HOW: Zoom meeting
AUDIENCE: This event is open to the public. All welcome!
PRESENTERS: Anglin Dent and Jethro Kwong
Anglin Dent
Department of Laboratory Medicine & Pathobiology, Temerty Faculty of Medicine
Presentation: Development and Potential Applications of Unsupervised Digital Pathology Tools in the Resolution of Tumor Heterogeneity
The widespread adoption of developed AI tools in clinical practice is often limited by the extensive manual annotation and training required for traditional supervised classification tasks. To overcome this limitation, our lab has focused our efforts on the development of generalizable unsupervised AI workflows capable of resolving pertinent intra- and inter-tumoral differences. By leveraging the human-like ability of AI to extract knowledge and discover underlying patterns within data, we have successfully developed a generalizable workflow that can be applied to challenges across cancer sub-classifications. I am very excited to share how our lab successfully developed these unsupervised AI tools and discuss some of their potential clinical applications.
• Anglin Dent on Google Scholar
• Q&A with Anglin Dent
_________________________________
Dr. Jethro Kwong
Division of Urology, Department of Surgery, Temerty Faculty of Medicine
Presentation: Development and external validation of an explainable machine learning model to predict risk of side-specific extraprostatic extension in men with prostate cancer
Radical prostatectomy, or surgical removal of the prostate, is one of the most common operations that urologists perform for patients with localized prostate cancer. A significant challenge during surgical planning is striking a balance between satisfactory oncological (ie: reducing the risk of positive surgical margins or leaving some cancer behind) and functional outcomes (ie: maintaining urinary continence and erectile function). In clinical practice, urologists often use nomograms to predict the risk of extraprostatic extension (ie: cancer that has spread outside the prostate capsule) to inform the decision to perform nerve-sparing, which will largely impact functional outcomes. However, current methods are either not specific enough (ie: predicts overall risk, not side-specific risk) or rely on specialized imaging which is not routinely available in Canada.
We have developed a novel explainable machine learning tool to provide accurate, explainable, and personalized predictions of side-specific extraprostatic extension using data that is readily available as part of the standard diagnostic workup for prostate cancer. Our model provides a comprehensive tool to facilitate patient counselling and tailored surgical strategy for this critical decision point.
• Jethro Kwong on Google Scholar
• Twitter: @jethro_kwong
• Q&A with Dr. Jethro Kwong
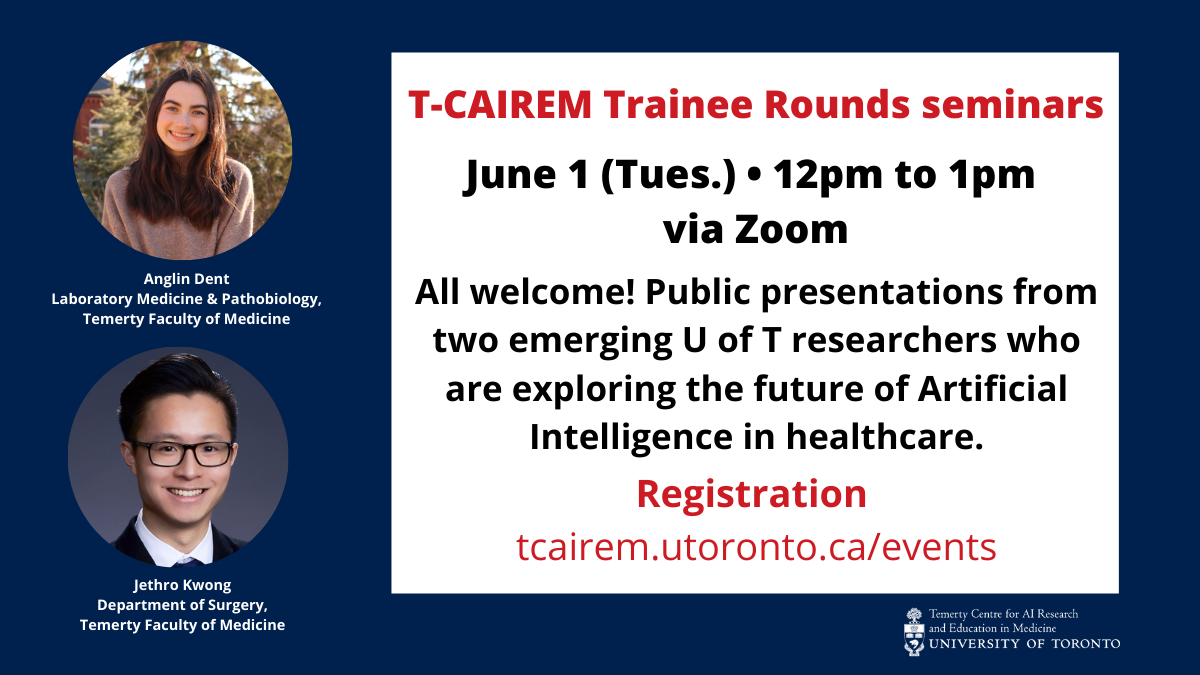