Main Second Level Navigation
Trainee Rounds: Phoenix Yu Wilkie and Sébastien Quetin
DATE: June 21, 2023 (Wed.)
TIME: 12pm to 1pm ET
METHOD: Zoom
PRESENTERS: Phoenix Wilkie and Sébastien Quetin
This event has passed.
Phoenix Wilkie
Graduate student, Division of Neurology, Department of Medicine, University of Toronto
TITLE: Creating better whole slide image datasets: Quality control detection of out-of-focus patches in digital pathology
ABSTRACT: We created a user-friendly GUI that clinicians can easily use for focus quality detection. This streamlines digital pathology workflows and improves downstream machine-learning tasks. We have trained an accurate OOF model using WSI patches derived from 52 different tissue areas and stained with a variety of stains. To augment publicly available curated datasets with manually annotated OOF patches, we developed a method of generating realistic synthetic out-of-focus patches. Our model was validated using a publicly available, human-labelled dataset of natural OOF patches and achieved high accuracy. Our user-friendly GUI evaluates a WSI in 30 seconds on a consumer-grade GPU.
ABOUT: Phoenix Yu Wilkie is working towards a PhD in the Medical Biophysics program at the University of Toronto. She completed a Bachelor of Computer science specialisation in Biomedical Computing at Queen’s University. Her main research focus is applying weakly supervised learning to predict ductal carcinoma in situ recurrence in patients. Her previous research included creating segmentation algorithms for CT and MRI, rapid 3D printing prototyping of medical devices, and 3D model development for facial orthotics and transtibial leg prosthetics at the University of Toronto.
MORE INFORMATION:
Sébastien Quetin
PhD Candidate, Medical Physics Unit, Department of Oncology, McGill University
TITLE: High precision Deep Learning based dose prediction in Brachytherapy
ABSTRACT: In high dose rate brachytherapy, dose in water is commonly used in a clinical setting to compute the dose absorbed by the body of patients. This approach allows for rapid dose calculation. Monte Carlo (MC) simulations are the gold standard to compute dose in a heterogeneous geometry like patient-specific absorbed dose. However, their extended computation time prevents their use in clinical settings. In this study, we demonstrate that Deep Learning can be used to predict dose with gold standard accuracy at the high resolution required in the clinic within a time acceptable for clinical use.
ABOUT: Sébastien Quetin is a PhD candidate in the Biological and Biomedical Engineering department of McGIll University. Before that, he attended INSA Toulouse, a five-year engineering school in the south of France where he specialized in Mathematics and Data Science. He is passionate about Artificial Intelligence and deep learning and is thrilled to apply them to Medical Imaging. He is working on automating several tasks in the brachytherapy workflow.
MORE INFORMATION:
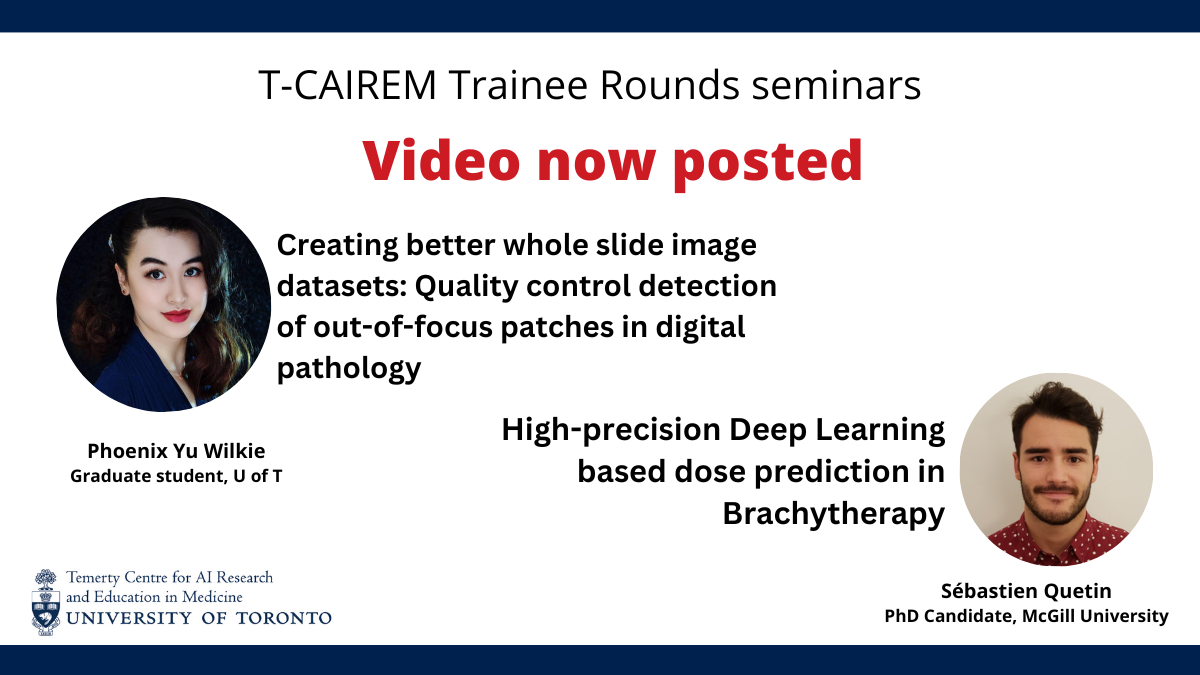