Main Second Level Navigation
Trainee Rounds: Ernest Namdar and Baijiang Yuan
DATE: June 25 (Tue.)
TIME: 12pm to 1pm ET
METHOD: Zoom
PRESENTERS: Ernest Namdar and Baijiang Yuan
Ernest Namdar
PhD candidate, Institute of Medical Science, Temerty Faculty of Medicine,
University of Toronto
TITLE: MRI-based Pediatric Low-Grade Neuroepithelial Tumor Molecular Biomarker Identification Pipeline; Towards Precision Medicine with Bi-institutional Data and Machine Learning
ABSTRACT: Pediatric Low-Grade Neuroepithelial Tumor (PLGNT) is the most common type of brain tumor in children. The current gold standard for PLGNT diagnosis is biopsy, which is invasive and may be infeasible, conditioned on tumor location. Imaging-based pipelines will eliminate the drawbacks. Previously, we showed that radiomics-based machine learning (ML) models are effective for determining the presence of BRAF fusion and BRAF V600E mutation in PLGNT. Aligned with precision medicine, we propose ML pipelines to achieve per-patient predictions. Our per-patient model inferences facilitate group-based performance analysis (e.g., performance for different institutes, genders, tumor locations, and time spans).
ABOUT: Ernest is a PhD candidate at the Institute of Medical Science, University of Toronto. He is also a senior data analyst at the Hospital for Sick Children (SickKids), a software engineering PEng holder, a Vector-Institute-affiliated graduate student, and an NVIDIA Deep Learning Institute’s AI ambassador in healthcare. Ernest is researching Pediatric Low-Grade Neuroepithelial Tumor (PLGNT), the most common brain tumor in children. He aims to replace the invasive and risky biopsy of the tumor tissue with imaging-based AI pipelines to determine molecular markers of PLGNT, which are decisive for successful early diagnosis and individualized treatment strategy selection.
Baijiang Yuan
PhD-track trainee, Institute of Medical Science, Temerty Faculty of Medicine,
University of Toronto
TITLE: Machine Learning Predicts Symptom Dynamics During Cancer Treatment
ABSTRACT: Symptom deterioration due to cancer and its treatment significantly burdens quality of life and poses challenges for healthcare providers, requiring complex cost interventions. The symptoms experienced by people with cancer are highly variable, influenced by both the nature of their treatment regimens and individual responses. Predicting and deeply understanding symptom trajectories yields benefits for people with cancer, clinicians, and the healthcare system. Our preliminary results suggest machine learning systems trained using longitudinal EMR data can accurately predict a wide range of symptom deterioration and provide downstream benefits.
ABOUT: Baijiang (Noke) Yuan is currently an MSc. to Ph.D track student at IMS (Institute of Medical Science). My research area is applied science, particularly using AI in healthcare to improve patient care.
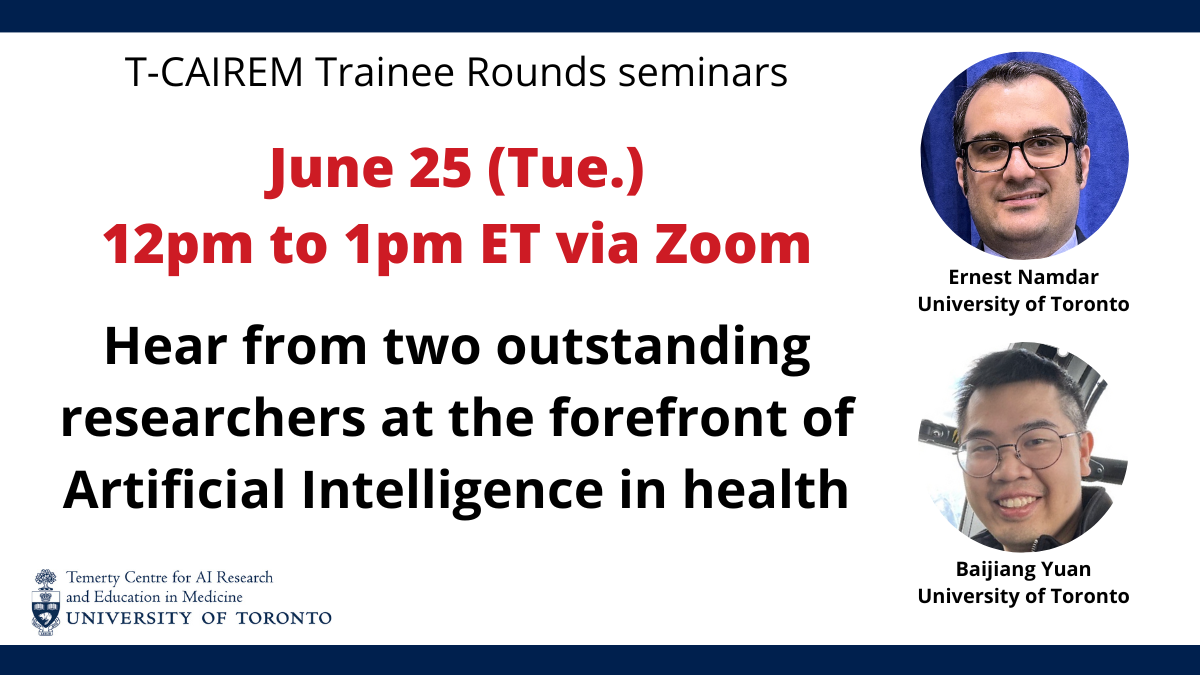