Main Second Level Navigation
Trainee Rounds: Arjun Balachandar & Mohammad Ali Khan
DATE: June 7, 2023 (Wed.)
TIME: 12pm to 1pm ET
METHOD: Zoom
PRESENTER: Dr. Arjun Balachandar
NOTE: Dr. Mohammad Ali Khan won't be able to present today, and his talk will be rescheduled.
Dr. Arjun Balachandar, MD
Division of Neurology, Department of Medicine, University of Toronto
TITLE: Automated Sleep Detection in Movement Disorders Using Deep Brain Stimulation and Machine Learning
ABSTRACT: Sleep disturbances in movement disorders can be debilitating. Deep Brain Stimulation (DBS) can treat motor symptoms, but treating sleep disorders requires detecting sleep. We recorded neural activity in various movement disorders in patients with DBS and created new machine-learning methods to detect sleep accurately.
ABOUT: Dr. Balachandar is a fourth-year Adult Neurology resident at the University of Toronto. He completed his undergraduate studies at McGill, then medical school at U of T. His research interests include neuromodulation, brain electrophysiology in movement disorders, and deep brain stimulation.
MORE INFORMATION:
Dr. Mohammad Khan, MD
Department of Family and Community Medicine, University of Toronto
TITLE: Machine Learning Quantification of Fluid Volume in Eyes with Retinal Vein Occlusion Undergoing Treatment with Aflibercept: The REVOLT study
ABSTRACT: To investigate the combined relationship between ischemia, retinal fluid, and layer thickness measurements with visual acuity outcomes for RVO patients and derive insights into disease pathology using machine learning. SS-OCT data were used to assess retinal layer thicknesses and quantify both intraretinal fluid (IRF) and subretinal fluid (SRF) using a deep learning-based, macular fluid segmentation algorithm for 49 treatment-naive eyes that were diagnosed with visual impairment due to central or branch RVO. Patients received three loading doses of 2 mg intravitreal aflibercept injections (IAI) and then were put on a treat-and-extend regimen. Image analysis was performed at baseline, three-month, and six-month follow-ups. Baseline OCT morphological features and fluid measurements were correlated using the Pearson correlation coefficient (PCC) to changes in BCVA to determine which features most impacted change in BCVA at 6 months. Areas of non-perfusion in OCTA images at baseline were also correlated with changes in BCVA at six months. A combined model of IRF volume, OPL and RNFL layer thicknesses, alongside ischemic indices, provides the best correlation to BCVA changes. Combined fluid and layer segmentation of OCT images provides clinically useful biomarkers for RVO patients.
ABOUT: Dr. Khan is enrolled in the Family Medicine program at the University of Toronto. Originally a trained data scientist working with TD Bank, he became interested in the applications of Artificial Intelligence to improve patient outcomes in a variety of medical fields. After completing his Master's degree in Statistics, he left his job at TD and enrolled in the MD program at McMaster University to better utilize analytical methods to aid patients.
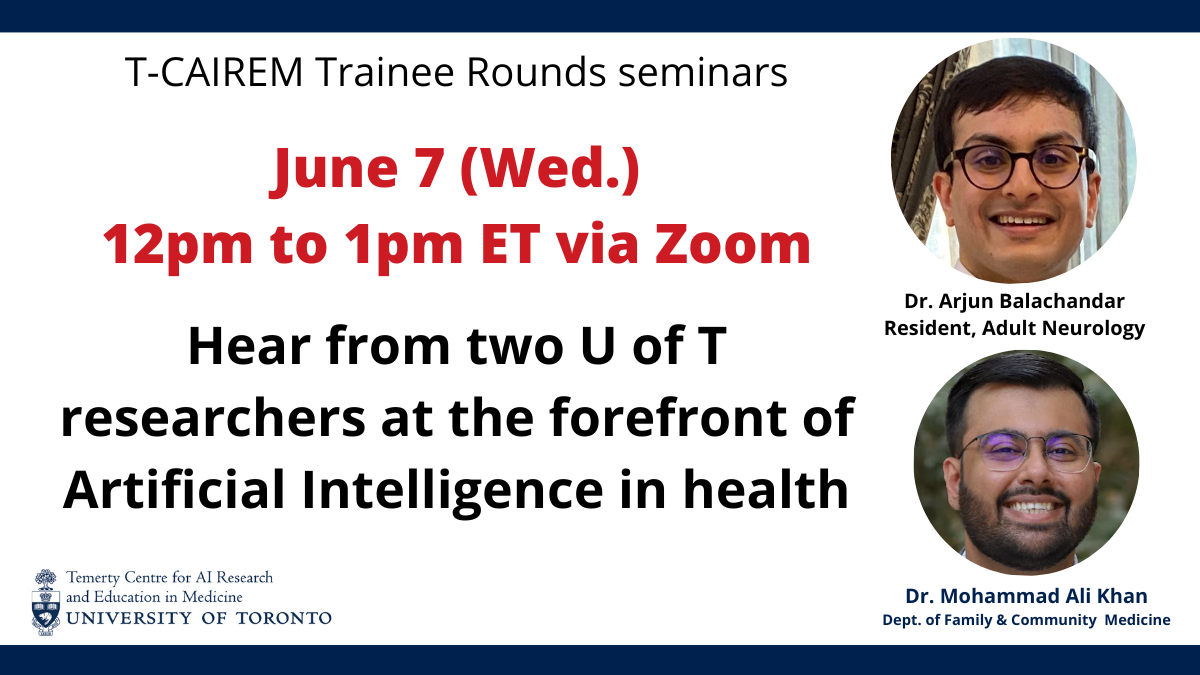