Temerty Centre Speaker Series: Finale Doshi-Velez
DATE: November 9, 2021 (Tuesday)
TIME: 12pm to 1pm
METHOD: Zoom webinar
AUDIENCE: This event is open to the public. All welcome!
NOTE: This event is CPD-accredited for physicians
Registration form
This event is now over. We will post a recording of the webinar here: https://tcairem.utoronto.ca/past-events
Biography
Finale Doshi-Velez is a Gordon McKay Professor in Computer Science at the Harvard Paulson School of Engineering and Applied Sciences. She completed her MSc at the University of Cambridge as a Marshall Scholar, her PhD from MIT, and her postdoc at Harvard Medical School. Her research interests are at the intersection of machine learning, healthcare, and interpretability. She heads the Data to Actionable Knowledge (DtAK) group at Harvard Computer Science which uses probabilistic methods to address many decision-making scenarios involving humans and AI. DtAK's work spans specific application domains (health and wellness) as well as broader socio-technical questions around human-AI interaction, AI accountability, and responsible and effective AI regulation.
Title of lecture
Reinforcement Learning in Healthcare: Applications and Validation
Abstract
Reinforcement learning (RL) has received a lot of attention as a way to train an agent or decision-support system to optimize for long-term outcomes in the face of uncertainty. In this talk, I'll discuss our journey toward developing RL agents to assist in hypotension management decisions for patients in the ICU. At every stage, our core question was: do we trust this agent? Each refinement of our approach reflects a step toward creating a system that not only appears to perform well but can be validated. I'll use this example from our work to discuss more broadly how to design and validate RL agents in the context of healthcare.
Learning objectives
1. Understand how to define a reinforcement learning problem in a health context (and when using reinforcement learning might be appropriate)
2. Understand, conceptually, how one might train a reinforcement learning agent given retrospective data
3. Understand the places where the training might go wrong and how to perform validation
Learn more
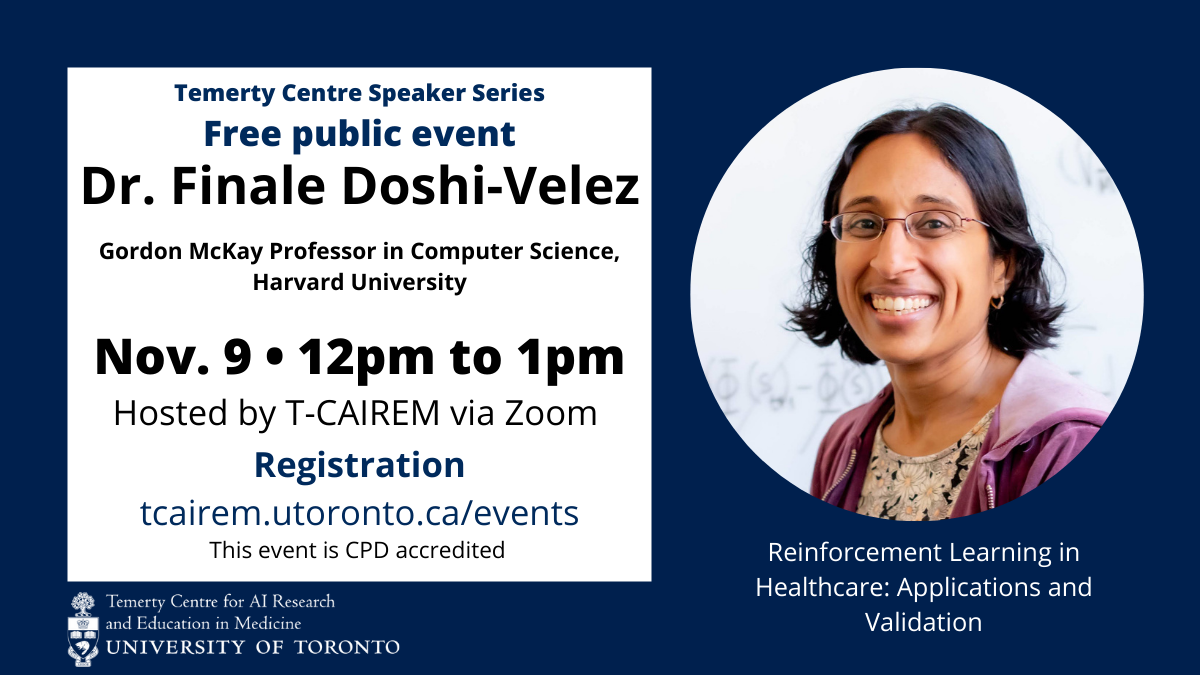