Main Second Level Navigation
Temerty Centre Speaker Series: Dr. Jenna Wiens
This event is now over. We'll post the video to our website as soon as we're able. Thanks to all who attended the event!
TIME: 12pm to 1pm
HOW: Zoom webinar
AUDIENCE: This event is open to the public. All welcome!
NOTE: This event is CPD-accredited for physicians
Associate Professor of Computer Science and Engineering (CSE) & co- Director of Precision Health
University of Michigan in Ann Arbor
Dr. Wiens' primary research interests lie at the intersection of machine learning, data mining,and healthcare. Wiens received her PhD from MIT in 2014, was named Forbes 30 under 30 in Science and Healthcare in 2015, received an NSF CAREER Award in 2016, was named to the MIT Tech Review's list of Innovators Under 35 in 2017, and was recently awarded a Sloan Research Fellowship in Computer Science.
Affiliations: Department of Electrical Engineering and Computer Science, University of Michigan, Ann Arbor
Title of lecture: From Diagnosis to Treatment - Augmenting Clinical Decision Making with Artificial Intelligence
Abstract: Though the potential of artificial intelligence (AI) in healthcare warrants genuine enthusiasm, meaningful impact will require careful integration into clinical care. AI tools are susceptible to mistakes and rarely capable of capturing all of the nuances pertaining to a complex clinical situation. Thus, we propose approaches designed to augment, rather than replace, clinicians during clinical decision making. In this talk, I will highlight two related research directions in which we propose i) a transfer learning approach for mitigating potentially harmful shortcuts when making diagnoses and ii) a novel reinforcement learning approach for matching patients to treatments. In summary, there’s a critical need for machine learning in healthcare; however, the safe and meaningful adoption of these techniques will require collaboration between clinicians and AI.
Learning objectives:
1. Recognize the potenial of deep learning approaches to exploit shortcuts.
2. Gain familiarity with techniques based on transfer learning for mitigating shortcuts.
3. Understand the difference between reinforcement learning in which we map states to actions and reinforcement learning with set-valued policies in the context of selecting treatments.
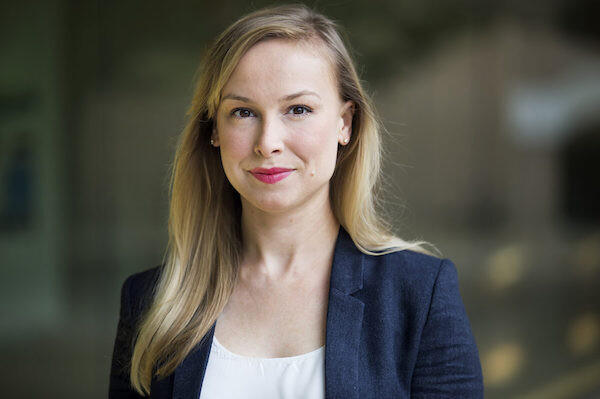