Breadcrumbs
2024 DSI/T-CAIREM Catalyst Grants
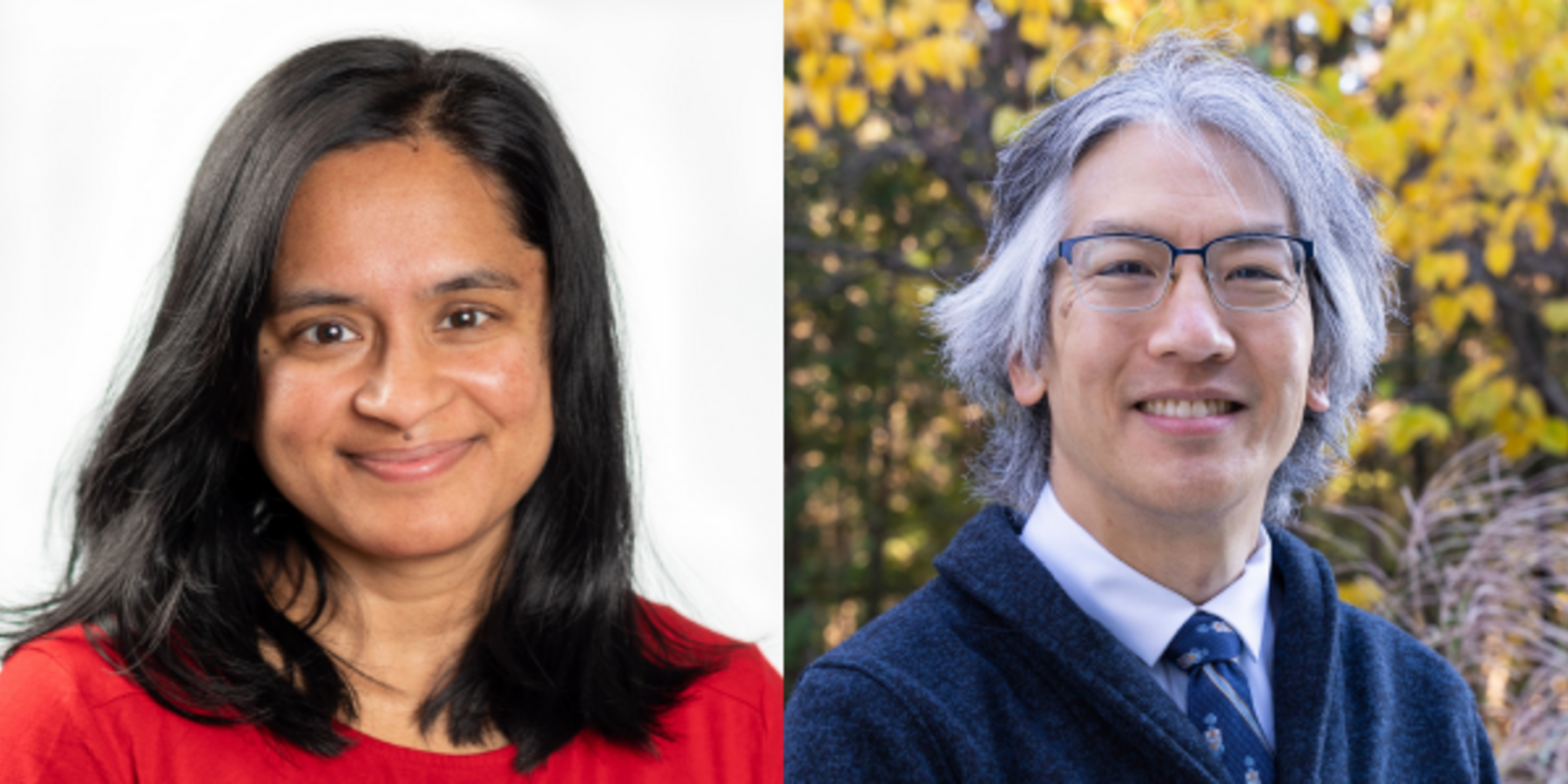
The Data Sciences Institute (DSI) and the Temerty Centre for AI Research and Education in Medicine (T-CAIREM) at the University of Toronto are pleased to announce two winners of the 2024 Catalyst Grant Awards. Each grant provides up to $100,000 in seed funding for multidisciplinary researchers forming Collaborative Research Teams (CRTs) that are developing novel statistical or computational tools that address important societal needs.
Dr. Sharmistha Mishra
Dr. Sharmistha Misra is an infectious disease physician and mathematical modeler. She holds a Tier 2 Canadian Research Chair in Mathematical Modeling and Program Science,
Proposal Abstract
“Examining biases due to confounders and colliders in observational health data using individual-based simulation models”
Large, health-administrative data are the bedrock of observational studies in medicine, including of both non-communicable and infectious diseases, to identify risk factors and intervention effects using statistical and machine learning models.
However, two types of biases, residual confounding and collider bias, can compromise the validity of inference on target parameters (e.g., intervention effect) from these data. These biases can produce results that mislead policy decisions, resource allocation, and clinical management.
We seek to (1) compare the direction and magnitude of these biases under various epidemiological conditions; and (2) examine the utility of different observational study designs in reducing these biases.
We will generate synthetic datasets (with the “true” causal effect, confounders, colliders as inputs) using two individual-based simulation models: (a) time-constant incidence of a generic non-communicable disease; and (b) time-varying incidence of an infectious disease outbreak, with a dynamic, sexual network model using sexual behaviour and health-administrative data to replicate the 2022 Monkeypox outbreak in Toronto, Canada.
We will sample from the synthetic datasets to mimic different observational study designs and compare the direction and magnitude of biases. This work has the potential for applicability across health conditions by helping to improve validity in estimating risks and intervention impact.
Dr. Tom Chau
Dr. Chau and his PRISM lab focus on access innovations such as the discovery, design, and evaluation of novel pathways by which children and youth can communicate or interact with their environment in the absence of functional speech and gestures.
Proposal Abstract
“Decoding unintelligible speech: a conversational context-aware assistive technology for children with complex communication needs"
Children with complex communication needs (CCN) often retain the ability to vocalize. They prefer this communication modality over all others, although the sounds tend to be unintelligible to unfamiliar communication partners.
There are currently no assistive technologies that can accurately decode their speech sounds, which may consist of multilingual and non- word utterances. Consequently, children with CCN remain excluded from full participation in education, society, and eventual employment.
This project will catalyze the development of a machine-learning pipeline to decode the unintelligible speech of these children. We will initially leverage an existing annotated audio-video dataset of unintelligible speech samples collected by the co-PI’s lab. We will also begin the creation of an audio-video-neurophysiological dataset of communicative interactions of children with CCN.
This novel pipeline will include intent extraction from communication partner speech. We will investigate both traditional machine learning entailing feature engineering and classification, as well as deep recurrent network approaches.
This project will provide training opportunities for students with a multiplicity of intersectional identities, particularly those with disability. This project will accelerate the impact of data sciences in the rehabilitation and biomedical engineering disciplines to drive positive social change for children with CCN.